If generative AI models are to continue to expand, they will need high-quality, human-created training data say scientists who found that using AI content corrupts the output.
Researchers from the University of Cambridge discovered that a cannibalistic approach to AI training data quickly leads to the models churning out nonsense and could prove to be a fork in the road for the rapid expanse of AI.
The team used mathematical analysis to show the problem that affects large-language models (LLMs) like ChatGPT as well as AI image generators like Midjourney and DALL-E.
The study was published in Nature which gave the example of building an LLM to create Wikipedia-like articles.
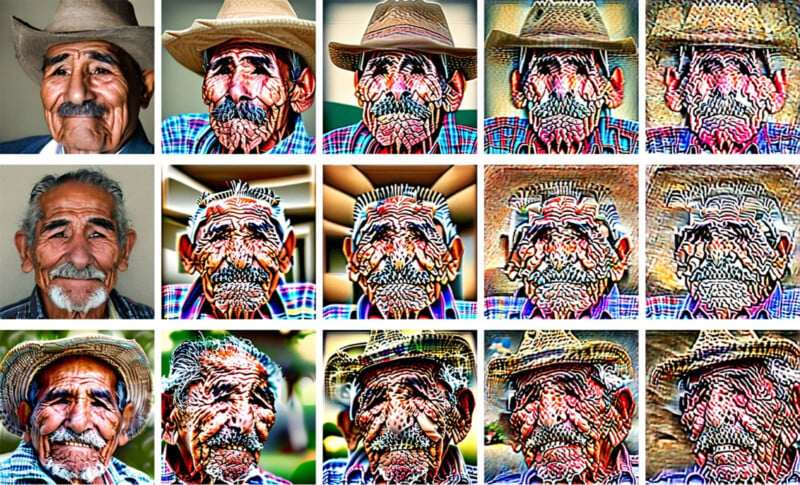
The researchers say that it kept training new iterations of the model on text produced by its predecessor. As the synthetic data polluted the training set, the model’s output became nonsensical.
For example, in an article about English church towers, the model included extensive details about jackrabbits. Although that example is at the extreme end, any AI data can cause models to malfunction.
“The message is, we have to be very careful about what ends up in our training data,” says co-author Zakhar Shumaylov, an AI researcher at the University of Cambridge, UK. “Otherwise, things will always, probably, go wrong”.
The team tells Nature they were surprised by how fast things started going wrong when using AI-generated content as training data.
Hany Farid, a computer scientist at the University of California, Berkeley, who has demonstrated the same effect in image models, compares the problem to species inbreeding.
“If a species inbreeds with their own offspring and doesn’t diversify their gene pool, it can lead to a collapse of the species,” says Farid.
Shumaylov predicts that this technological quirk means that the cost of building AI models will increase as the price of quality data increases.
Another issue for AI companies is that as the open web — where most scrape data from — is filled up with AI content it will pollute their resource forcing them to rethink.
However, few copyright holders will shed a tear at this problem faced by AI companies. Artists, photographers, and content creators of all stripes have been up in arms over the tech industry’s brazen use of their work to build AI models.
Image credits: M. Boháček & H. Farid/arXiv (CC BY 4.0)