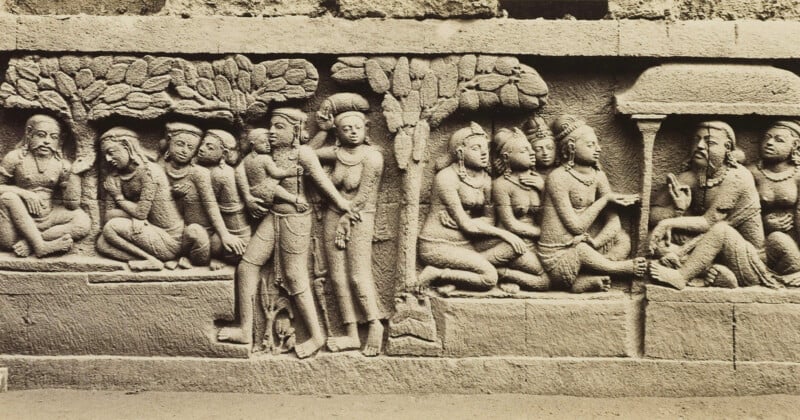
Scientists have created a 3D model of a buried relief sculpture by using a photo taken in the 1800s and novel AI technology.
The researchers from Ritsumeikan University in Japan developed a neural network capable of looking at a standard 2D photograph of a 3D object and producing a digital reconstruction in 3D.
In this case, the team looked at a photo showing figures carved into stone, known as a relief, that is buried in Borobudur Temple in Indonesia — a UNESCO World Heritage Site and the world’s largest Buddhist temple compound.
According to Gizmodo, the black-and-white photo was taken 134 years ago of a relief that was only temporarily exposed because of reconstruction work. Photographs were taken of the relief before they were buried again and have been for the last century.
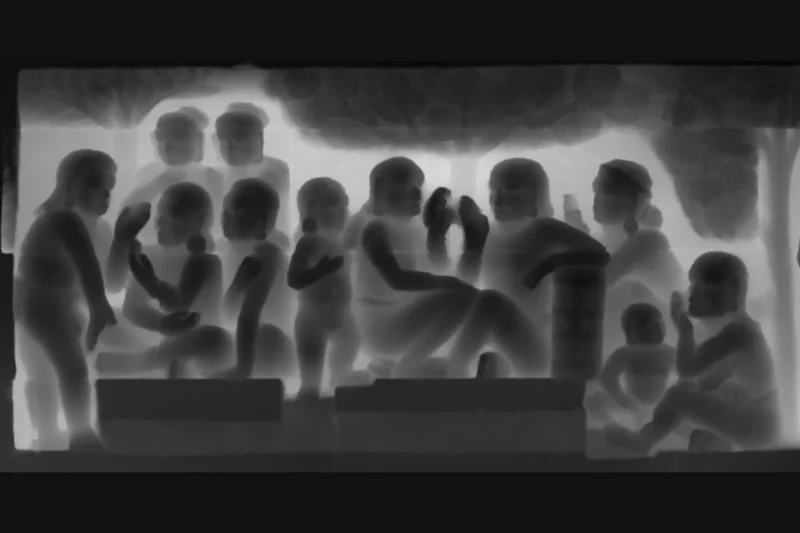
Other research teams have tried making 3D reconstructions but couldn’t because of the compression of depth values.
“Previously, we proposed a 3D reconstruction method for old reliefs based on monocular depth estimation from photos. Although we achieved 95% reconstruction accuracy, finer details such as human faces and decorations were still missing,” explains Professor Satoshi Tanaka from Ritsumeikan University.
“This was due to the high compression of depth values in 2D relief images, making it difficult to extract depth variations along edges. Our new method tackles this by enhancing depth estimation, particularly along soft edges, using a novel edge-detection approach.”
The team’s multi-modal neural networks perform three tasks: semantic segmentation, depth estimation, and soft-edge detection, which work together to enhance the accuracy of 3D reconstruction.
The core strength of the network lies in its depth estimation, achieved through a novel soft-edge detector and an edge-matching module. Unlike the conventional binary edge classification, the soft-edge detector treats edge detection of relief data as a multi-classification task.
Edges in relief images not only represent changes in brightness but also variations in curvature, known as “soft edges”. The soft-edge detector determines the degree of “softness” of these edges in relief images, enhancing depth estimation.
The edge matching module comprises two soft-edge detectors that extract multi-class soft-edge maps and a depth map, from an input relief photo. By matching and detecting differences between the two maps, the network focuses more on the soft-edge regions, resulting in more detailed depth estimation.
Finally, the network optimizes a dynamic edge-enhanced loss function, which includes loss from all three tasks, and produces clear and detailed 3D images of reliefs.
You can read the team’s paper here.
Image credits: Pan et al. 2024