AI image generators are controversial because they were largely built on copyrighted works of artists and photographers who did not consent. But how would they feel if corrupted versions of their work were used instead?
A research team led by the University of Texas has come up with a model called Ambient Diffusion which they claim “gets around” the issue of copyright and AI image generators by feeding the model images that have pixels missing — in some cases as much as 93%.
“Early efforts suggest the framework is able to continue to generate high-quality samples without ever seeing anything that’s recognizable as the original source images,” reads a press release.
The project started by training a text-to-image model with images that had pixels partially masked but for Ambient Diffusion the team began experimenting with corrupting images with other types of noise.
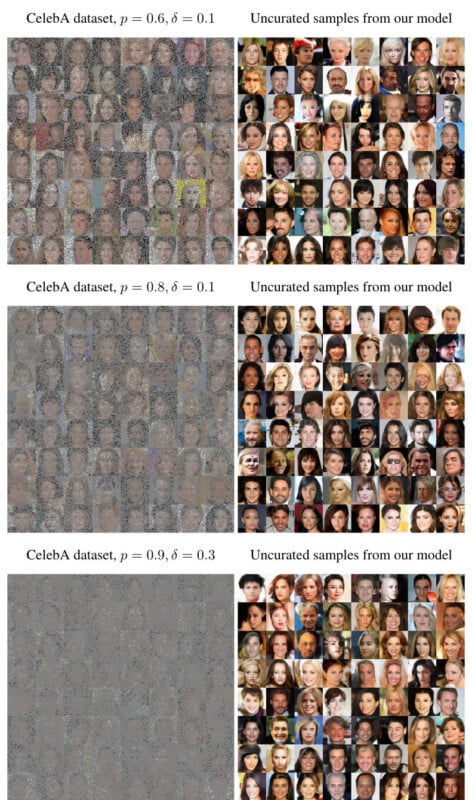
slightly worse as we increase the level of corruption, but we can reasonably well learn the distribution even with 91% pixels missing (on average) from each training image.
The first diffusion model was trained with a clean set of 3,000 images of celebrities which spat out “blatant copies” of the training data.
However, when the researchers began corrupting the training data, randomly masking up to 90 percent of the pixels, the image generator still created “high quality” images of humans but the resulting pictures didn’t look like any of the real-life celebrities.
“Our framework allows for controlling the trade-off between memorization and performance,” says Giannis Daras, a computer science graduate student who led the work. “As the level of corruption encountered during training increases, the memorization of the training set decreases.”
The researchers say that the model didn’t just spit out pictures of noise as some may have expected, although the performance still changed with the quality of the output worsening the more the photos were masked.
“The framework could prove useful for scientific and medical applications, too,” adds Adam Klivans, a professor of computer science, who was involved in the work. “That would be true for basically any research where it is expensive or impossible to have a full set of uncorrupted data, from black hole imaging to certain types of MRI scans.”
Members of the University of California, Berkeley, and MIT were also part of the research team. The paper can be read here.
Image credits: Giannis Daras, Kulin Shah, Yuval Dagan, Aravind Gollakota, Alexandros G. Dimakis, Adam Klivans.