Much of the focus on the potential hazards of generative AI technology has understandably been on how it impacts artists and copyright ownership, and how it could affect people’s jobs and wages. New research shows that the dangers of generative AI go much further and may be catastrophic for the environment.
As seen on Engadget, researchers from the AI startup Hugging Face worked alongside scientists at Carnegie Mellon University in Pittsburgh to determine the carbon footprint of different AI-generated content.
Researchers determined that generating text requires less energy than synthesizing an image. Although this could be reasonably filed under, “Of course it does,” what is novel and important is that the team determined precisely how much energy different generative tasks consume, and the results are rather startling.
A straightforward task such as classifying text using AI generates roughly between 0.2 and 0.5 grams of carbon dioxide emissions per 1,000 queries. However, image generation creates more CO2 emissions than that by a factor of up to 2,000. Put another way, asking a generative AI platform to make 1,000 images can produce up to 1,000g of carbon dioxide.
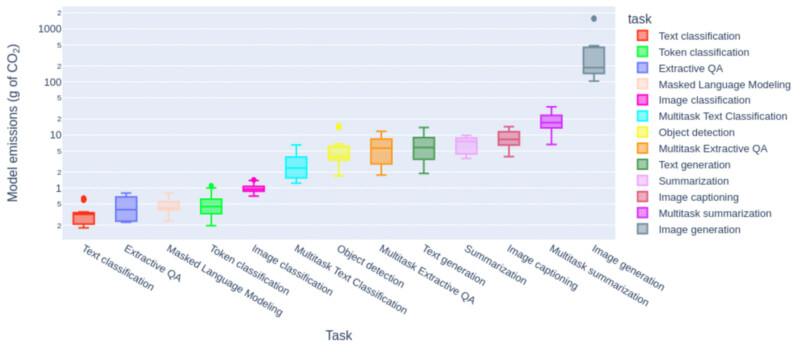
“We find that multi-purpose, generative architectures are orders of magnitude more expensive than task-specific systems for a variety of tasks, even when controlling for the number of model parameters. We conclude with a discussion around the current trend of deploying multi-purpose generative ML systems, and caution that their utility should be more intentionally weighed against increased costs in terms of energy and emissions,” write the researchers in a new paper.
Regarding energy consumption, the average cost of 1,000 text classification queries, the least costly of the 10 common AI tasks the team measured, is 0.002 kWh. Meanwhile, image generation, by far the costliest of the tested tasks, requires 2.907 kWh per 1,000 instances. The difference between the two extreme ends is a factor of more than 1,450.
2.907 kWh may not sound like much. However, put another way, the energy it takes to charge a completely depleted Telsa Model 3 battery fully is 50 kWh. So, generating about 17,200 images using AI requires the same electricity as charging a car.
Although it is difficult to determine precisely how many images different AI platform users create each day, if a popular platform like DALL-E is similarly taxing as the research team’s model, DALL-E users use the energy it takes to charge nearly 2,000 electric cars daily to generate images. Add in platforms like Midjourney, Adobe Firefly, and other apps and services, and it’s easy to imagine a much higher electricity demand.
The researchers determined that the most carbon-intensive image generation model, a form of Stable Diffusion, generates 1,594 grams of CO2 per 1,000 inferences. This is like driving the average gas-powered car for about 4.1 miles. Four miles doesn’t sound like much, but that is only for 1,000 inferences, and the totals add up extremely quickly.
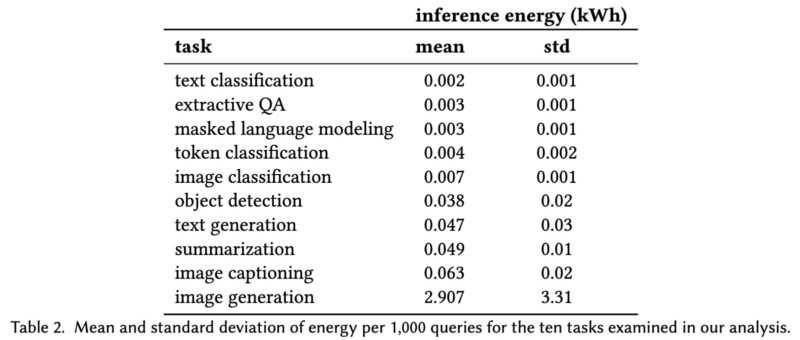
That said, the team is quick to note that while that is the most extreme case right now, there is quite a bit of variance across different image generation models concerning energy consumption and emissions. This could prove extremely important as legislators grapple with AI’s real-world costs.
Another factor worth considering is the extreme cost of training and deploying AI models. The researchers observe that it is very challenging to determine the cost of model training as the data required to do so is rarely published. However, training is known to be “orders of magnitude more energy- and carbon-intensive than inference,” or using a model. This is yet another consideration for lawmakers.
While the researchers faced numerous challenges, including limited datasets and difficulty determining the precise cost of every factor of developing, training, and using various AI models, the work has a few critical implications.
For starters, the research helps fill a massive void in understanding artificial intelligence technology’s energy demands and environmental costs. It has created the necessary framework to begin understanding and tackling these questions.
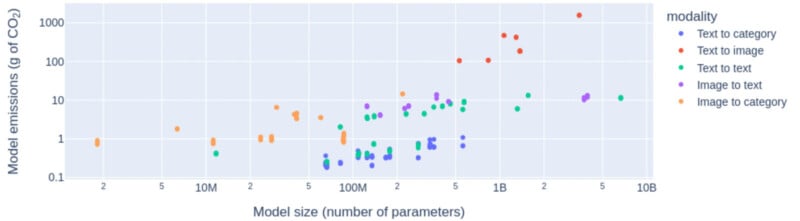
The study also helps contextualize one of the most important and underappreciated aspects of AI models, their insatiable appetite for energy and the severe environmental repercussions of their operation, which remains almost entirely unchecked.
Earlier this year, discussing how AI itself may help humans overcome the ever-increasing climate crisis, Columbia University asked, “Because of AI’s capabilities, there are many ways it can help combat climate change, but will its potential to aid decarbonization and adaptation outweigh the enormous amounts of energy it consumes? Or will AI’s growing carbon footprint put our climate goals out of reach?”
As AI becomes more popular, and especially as extremely resource-intensive generative video models continue to proliferate, training new models will continue to be a significant energy consumer, and, thus, a generator of carbon emissions. The latest study by Hugging Face and Carnegie Melon shows that as training parameters increase, energy demands get larger in turn.

2019 research at the University of Massachusetts Amherst found that training a single AI model could emit more than 626,000 pounds of CO2, which is the same as five cars over their lifetime.
AI models have become much more sophisticated since 2019. A more recent study showed that training GPT-3 with 175 billion parameters required 1,287 MWh of electricity and emitted more than 550 metric tons of carbon, which is like driving 112 typical gasoline-powered cars for a year.
When contextualizing the environmental cost of training, deploying, and using AI models, it is essential to consider the benefits of these technologies, too. Large language models have helped develop life-saving vaccines and are integral in the fight against many diseases, for example. There is no need to throw the baby out with the bathwater and lose these benefits.
However, as ongoing research demonstrates, AI companies must consider their energy consumption and carbon footprints, which requires that this data be tracked and made accessible. This becomes ever more vital as the climate crisis worsens and AI becomes increasingly more integrated into society. Ideally, legislation should properly regulate AI in a meaningful way that considers the larger human impact of AI, including its environmental effects.
Image credits: Figures and tables come from “Power Hungry Processing: Watts Driving the Cost of AI Deployment?” by Alexandra Sasha Luccioni and Yacine Jernite (Hugging Face, Canada/USA), Emma Strubell (Carnegie Mellon University, Allen Institute for AI, USA). The featured image in this article was generated using AI and subsequently offset, at least in earnest effort, through the financial support of a reforestation organization.